Transforming Ophthalmology with AI-Generated Diagnostic and Therapeutic Recommendations
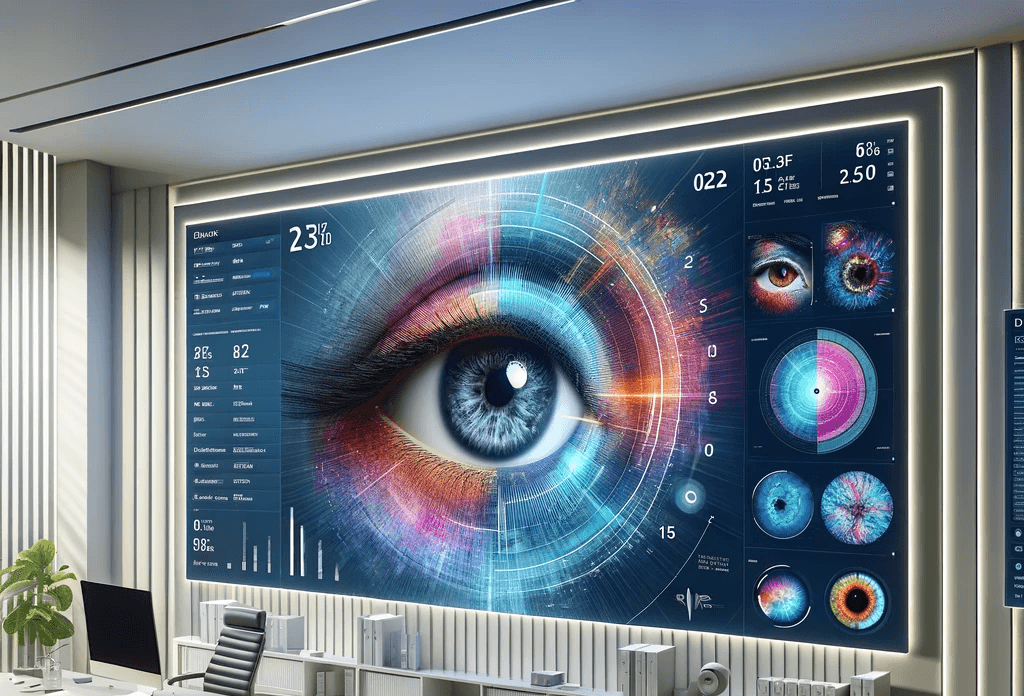
Introduction
In the past decade, the field of ophthalmology in general and vitreoretinal disease in specific, has witnessed tremendous strides in both diagnostic imaging techniques and novel treatments for blinding eye diseases. These advancements put us in a far better position to serve our patients, but also generate a significantly higher amount of clinical and imaging data for every visit. This poses unique challenges in patient care with regards to data collection, analysis and treatment customization. Ideally and hypothetically, for optimum patient care, a healthcare provider should make the best medical decision by compiling clinical history, examination data, laboratory testing and imagining results and also, factor in other variables such as gender, social habits, family history, race and ethnicity. It is rather inconceivable to assume that a provider can systematically gather and analyze all these data points and come up with a timely medical conclusion while caring for a patient. There are numerous examples of human errors in medical practices and it will only get worse when providers are dealing with a higher number of patients and more medical data. This is perhaps more challenging when dealing with the pediatric population, telehealth or remote patient screening.
Another major future challenge in healthcare is the disparity between the growth rate of the patient population versus providers. With an aging population, the number of patients will grow exponentially that leads to limited access to healthcare. Prime example is diabetic patients with an estimated total number of 86 million in the United States and 1.3 billion worldwide by 2050 who will need eye screening for diabetic retinopathy. With the current eyecare practice approaches and without any pivot, it will be impossible to see this many patients and provide care for them. The same is true for other blinding eye diseases that need constant monitoring.
As an ophthalmologist with a deep understanding of machine learning (ML) and the latest large language models (LLM), I am excited to introduce the next generation of AI-powered platforms aimed at revolutionizing the way eye conditions are screened, diagnosed and treated. Our vision is to create an innovative and comprehensive AI platform that leverages the power of ML and LLMs to enhance the accuracy, efficiency, and accessibility of ophthalmic healthcare. By harnessing cutting-edge technologies, we aim to bridge the gap between traditional medical practices and the limitless possibilities presented by AI. This white paper serves as a blueprint for our project, outlining the reasons behind our initiative and detailing how we plan to achieve our goals.
The Need for Innovation in Ophthalmology
Historically, eye imaging has been an inseparable part of day to day practice of Ophthalmology. Nowadays, with the advent of advanced imaging technologies such as OCT and ultrawide field fundus cameras, eye imaging is more readily accessible. Additionally, our AI MVP has evolved to an acceptable level of accuracy and reproducibility that can analyze imaging data and generate accurate and detailed medical reports. This provides a huge opportunity to circumvent many obstacles in patient care in Ophthalmology. Here are some of the major areas that our platform will have a tremendous impact:
Limited Access to Specialists:
A shortage of ophthalmologists, nationally and internationally, leads to long wait times for appointments and limited access to eye care, especially in remote and underserved areas. For instance, while the United States has about 50 ophthalmologists per 1 million people, Sub-Saharan Africa faces a severe shortage with only 2 ophthalmologists per 1 million, highlighting a global disparity in eye care resources (source). The access issue also exists in neonatal ICUs for screening of retinopathy of prematurity in newborns. In the grand scheme of things, the worldwide number of diabetic patients will disproportionately grow to a staggering 1.3 billion by 2050 while the health system growth will lag behind. Therefore, the need for an automated, accurate, and fast screening system is prudent and indeed necessary.
Diagnostic Accuracy:
While ophthalmologists are highly skilled, the accuracy of diagnosis can vary, and errors can have significant consequences for patients' vision and quality of life. This is more problematic for patients who live in remote areas, and only have access to providers with limited or unrelated training who are responsible for their care. Our AI-powered platform will immensely improve patient outcomes by providing quick and accurate diagnosis as well as medical recommendations. This will have significant economical benefits both for the patient and society.
Evolving Treatment Options:
The field of ophthalmology is constantly evolving, with new treatment options for complex eye diseases like age-related macular degeneration (AMD) emerging steadily. However, this makes it challenging for eye care providers to stay fully up-to-date and determine the most suitable treatment protocol tailored to each patient based on their clinical profile and disease attributes. For instance, in dry AMD, there is tremendous interest surrounding anti-complement antibody treatments to slow disease progression. By mining intricate patterns in clinical data and retinal imaging our system can identify AMD candidates likely to respond better to emerging complement inhibitors, while considering comorbidities, risk factors and expected adverse events.
Data Overload:
The amount of medical data generated daily is overwhelming, making it difficult for ophthalmologists to keep pace with the ever-increasing volume of information. Patients with advanced retinal diseases have 6-10 clinic visits in a year and go through a variety of multimodal imaging including OCT, IVFA, fundus color photos as well as a detailed clinical examination. A mid size practice provides care for about 20 to 25 thousands patients a year. Therefore the amount of data generated is staggering and certainly impossible to process in a timely manner by humans on a day to day practice. AI-powered platforms are the only viable solutions for meta data analysis.
Personalized Medicine:
Tailoring treatment plans to individual patients' needs is a complex task that could benefit from more advanced data analysis and AI-driven insights.
Prognostication:
Determining patient prognosis is a complex matter that relies on understanding the intricate relationship between various patient information, clinical findings and testing. ML and LLMs seem to be the only way forward to analyze meta data sets, identify prognosis-worthy biomarkers and generate meaningful reports.
Our proposed AI-powered ophthalmic platform aims to address above mentioned challenges and revolutionize the field. Here's how we intend to achieve this:
The Solution: AI-Powered Ophthalmic Platform
The advent of multimodal Large Language Models (LLMs) marks a significant evolution. These advanced models blend the capabilities of language transformers, akin to GPT or LLaMA, with the image-processing proficiency of encoders like CLIP. This synthesis enables them to perform complex tasks such as interpreting and describing images or scenes in a manner that is relatable and understandable to humans. Currently, we are fine-tuning the recently released LLaVA 1.5, a multimodal model built on the LLaMA framework. Our focus is on enriching this model with an extensive dataset of retina images from over 3,000 patients, each meticulously labeled with specific diseases such as diabetic retinopathy and macular degeneration.
While our MVP is centered around fundus imaging and diagnosis, we aim to develop a much more comprehensive platform with the following capabilities:
1. Comprehensive Data Analysis
We will leverage machine learning algorithms to analyze vast datasets of ophthalmic images, patient histories, laboratory testing, medical imaging and clinical records. By doing so, we can:
• Enhance diagnostic speed and accuracy by identifying subtle patterns and anomalies that might be missed by the human eye.
• Provide ophthalmologists with decision making support tools to improve their efficiency, diagnostic capabilities and treatment planning, especially for rare or controversial cases.
• Predict disease progression and the likelihood of complications, enabling early intervention, optimized drug selection and tailored follow up plans.
a. Easy Access to Fundus Image Analysis
In the first phase, we will provide eye care providers with easy access to our platform, allowing them to upload fundus images for analysis and to receive AI generated second opinions. This will democratize access to expert insights and help improve diagnostic accuracy. Our AI system will assist ophthalmologists and optometrists by:
• Identifying subtle abnormalities in fundus images that might be overlooked.
• Providing comparative analysis with a vast database of images, ensuring a comprehensive evaluation.
• Offering suggestions for potential treatment options based on the analysis.
b. Advanced Image Analysis and Diagnostics
In the second phase, our platform will evolve to analyze a wider range of ophthalmic images, including OCT scans, fluorescein angiography, and more. By expanding our dataset and leveraging machine learning capabilities, we aim to provide not only diagnostics but also predictive insights. This will enable eye care providers to:
• Detecting complex conditions at earlier stages, prompting earlier treatment and increasing the success of treatment.
• Receiving treatment plan recommendations tailored to individual patient needs.
• Accessing a wealth of historical data to inform their decisions and monitor disease progression.
c. Personalized Medicine and Predictive Protections
In the third and final phase, our platform will harness the metadata gathered from patients, including race, ethnicity, age, location, social habits, personal and family history as well as biological markers and lab testing, genetic analysis, etc. By integrating this information, we will create a holistic view of each patient, enabling the development of highly personalized treatment plans, prognostic and predictive protections. This phase will:
• Allow for the customization of treatment strategies based on individual demographics and genetic factors.
• Predict disease risks and complications, enabling proactive measures to protect patients' vision.
• Advance the field of ophthalmology by contributing valuable insights to research and epidemiology.
2. Telemedicine Integration
To address the issue of limited access to specialists, our platform will incorporate telemedicine capabilities. Health care providers from any discipline or even patients, regardless of their location, will be able to access AI-powered ophthalmic evaluation remotely for screening, reducing wait times and increasing accessibility. This will have huge social and economic impact and will improve quality of life for many patients in need.
3. Knowledge Transfer and Continuous Learning
We will implement a knowledge transfer system that ensures eyecare providers stay up-to-date with the latest research, treatment modalities, and guidelines. The platform will continuously learn from real-world cases and research publications, incorporating this knowledge into its recommendations.
4. Personalized Treatment Plans
Our AI system will generate personalized treatment plans by considering individual patient factors such as genetics, lifestyle, and medical history. This will optimize treatment outcomes and improve patient satisfaction.
5. Ethical Considerations
We are committed to adhering to strict ethical guidelines in the development and deployment of our platform. Patient privacy and data security will be paramount, and the platform will operate within the legal and regulatory framework.
Final Remarks
The convergence of ophthalmology, machine learning, and large language models presents a unique opportunity to transform eye healthcare. Our AI-powered ophthalmic platform aims to bridge the gap between limited access to specialists, the need for large scale patient screening and accurate diagnosis, and the demand for personalized treatment plans.